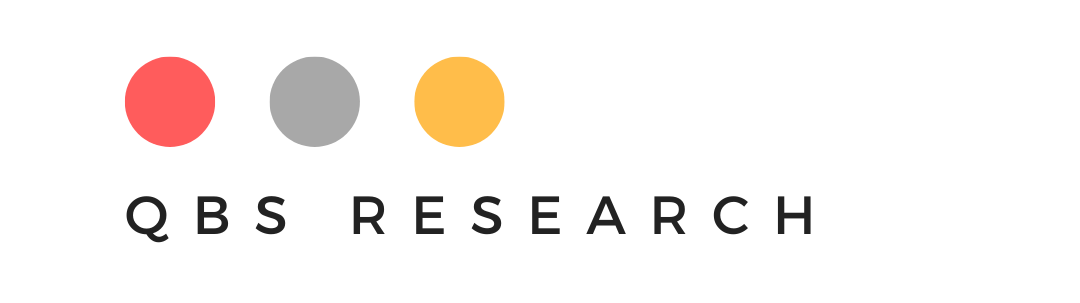
Background and Context
The Challenge of Detecting Informed Trading
Informed investors usually hide behind uninformed order flow, making their trading activities difficult to identify with traditional measures.
Machine Learning Approach
The researchers trained Gradient Boosted Trees algorithms on known informed trades to develop a new measure called Informed Trading Intensity (ITI).
Data and Methods
The study used Schedule 13D trades, insider trades, and short-selling data from 1993-2019, analyzing 41 variables related to liquidity, volume, volatility, and returns.
ITI Outperforms Traditional Measures in Detecting Informed Trading
- ITI detects Schedule 13D trading with more than twice the explanatory power of standard liquidity measures.
- The R-squared of 9.86% for ITI alone is substantial given informed investors actively hide their trades.
- ITI's effectiveness has remained stable over time while traditional measures' effectiveness has declined.
Volume and Volatility Interaction Crucial for Identifying Informed Trading
- ITI is highest when trading volume is high but volatility is low, contrary to some theoretical predictions.
- Volume-related variables are the most important factors in detecting informed trading activity.
- ITI captures nonlinear relationships that linear models would miss, highlighting the value of machine learning.
ITI Increases Before Major Information Events
- ITI spikes ahead of earnings announcements, especially those with large price impacts.
- Informed trading activity is detected 2-5 days before unscheduled news and M&A announcements.
- Post-announcement ITI remains elevated, suggesting informed investors capitalize on information processing advantages.
High ITI Days Show Significantly Less Price Reversal
- Returns on days with high ITI show reduced reversal, confirming they reflect informed trading.
- A two standard deviation increase in ITI reduces return reversal by approximately two-thirds.
- This pattern persists even when controlling for turnover, volatility, and liquidity effects.
ITI Predicts Higher Future Stock Returns
- Stocks with the highest ITI outperform those with the lowest ITI by 6.42% annually.
- Patient and impatient informed trading have different return implications, with impatient trading generating higher returns.
- The return predictability is consistent with buy-sell asymmetry rather than information risk pricing.
Contribution and Implications
- ITI offers researchers and practitioners a more effective tool to measure and analyze informed trading in financial markets.
- The data-driven approach reveals that informed trading patterns don't match current theoretical models, guiding future research.
- ITI distinguishes between patient and impatient informed trading, showing commonalities across different types of informed investors.
- The measure provides investors potential opportunities to profit from identifying stocks with high informed trading activity.
Data Sources
- Chart 1 was constructed using data from Table II, comparing R-squared values for detecting Schedule 13D trading.
- Chart 2 was developed based on the partial dependence plots in Figure 2 and decision tree analysis in Figure 3.
- Chart 3 visualizes data from Figure 4, showing ITI changes around earnings announcements and news events.
- Chart 4 is based on Table V's regression analysis of return reversal and ITI interactions.
- Chart 5 draws from Table XI, showing portfolio returns for stocks sorted by ITI measures.